Unlocking the Future of Artificial Intelligence: The Power of AI Infrastructure
In the ever-evolving landscape of artificial intelligence (AI), one crucial component stands out as the backbone of this technological revolution: AI infrastructure. This robust framework is not just a mere foundation; it's the engine that propels AI systems towards unprecedented heights of efficiency, scalability, and accuracy. As we stand at the threshold of the next generation of AI, it's essential to delve into the intricacies of AI infrastructure and understand its transformative impact on the industry.
The modern world is witnessing an AI-driven renaissance, where intelligent machines are being seamlessly integrated into various sectors, including healthcare, finance, education, and transportation. The proliferation of AI has been made possible by the cumulative efforts of researchers, engineers, and innovators who have worked tirelessly to develop and refine AI infrastructure. However, the journey to harness the full potential of AI infrastructure has been long and arduous, necessitating the development of specialized hardware, software, and expertise.
As AI infrastructure continues to evolve, it's clear that its influence will be felt across multiple domains. By providing a robust foundation for AI systems, AI infrastructure will play a pivotal role in shaping the future of industries, enhancing decision-making processes, and driving innovation. In this article, we will delve into the intricacies of AI infrastructure, exploring its key components, applications, and the future prospects of this rapidly evolving field.
The Anatomy of AI Infrastructure
AI infrastructure consists of several interconnected components that work in tandem to provide a seamless and efficient AI experience. These components can be broadly categorized into hardware, software, and data infrastructure.
Hardware Components
The hardware aspect of AI infrastructure includes specialized computing devices designed to handle complex AI algorithms and machine learning tasks. Some of the key hardware components of AI infrastructure include:
- Graphics Processing Units (GPUs): These specialized devices are designed to handle the intense computational requirements of AI algorithms, particularly those involving deep learning.
- Tensor Processing Units (TPUs): TPUs are custom-built chips designed specifically for machine learning and AI tasks, offering significant performance enhancements over traditional computing devices.
- High-Performance Computing (HPC) Clusters: These distributed computing systems are designed to provide massive parallel processing capabilities, making them ideal for complex AI workloads.
- Edge Devices: Edge devices, such as smartphones and smart home devices, enable AI algorithms to be deployed at the edge of the network, reducing latency and improving real-time decision-making.
Software Components
The software aspect of AI infrastructure includes the frameworks, libraries, and tools that enable AI systems to learn, reason, and interact with their environment. Some of the key software components of AI infrastructure include:
- Deep Learning Frameworks: Frameworks like TensorFlow, PyTorch, and Keras provide the necessary tools and libraries for building and deploying deep learning models.
- Natural Language Processing (NLP) Libraries: NLP libraries like NLTK, spaCy, and Stanford CoreNLP enable AI systems to understand and generate human language.
- Computer Vision Libraries: Computer vision libraries like OpenCV, Pillow, and scikit-image provide the necessary tools for image and video analysis.
- Cloud-Based AI Platforms: Cloud-based AI platforms like AWS SageMaker, Google Cloud AI Platform, and Microsoft Azure Machine Learning provide scalable and secure environments for AI development and deployment.
Data Infrastructure
The data infrastructure aspect of AI infrastructure encompasses the storage, processing, and management of large datasets required for AI system development and deployment. Some of the key data infrastructure components include:
- Data Warehouses: Data warehouses provide a centralized repository for storing and managing large datasets, making it easier to extract insights and patterns.
- Data Lakes: Data lakes provide a flexible and scalable repository for storing raw, unprocessed data, enabling AI systems to learn from diverse sources.
- Data Pipelines: Data pipelines orchestrate the flow of data between different sources, enabling real-time processing and analysis.
- Data Governance: Data governance ensures that data is accurate, complete, and secure, addressing the critical challenges of data quality and trust.
Applications of AI Infrastructure
AI infrastructure has far-reaching implications for various industries, enabling the development of innovative applications that were previously unimaginable. Some of the key applications of AI infrastructure include:
- Healthcare: AI infrastructure enables the development of personalized medicine, disease diagnosis, and predictive analytics for improving patient outcomes.
- Finance: AI infrastructure supports the creation of intelligent trading systems, risk analysis, and fraud detection, enabling financial institutions to make data-driven decisions.
- Education: AI infrastructure enables the development of intelligent tutoring systems, personalized learning platforms, and adaptive assessments, enhancing student outcomes.
- Transportation: AI infrastructure supports the creation of autonomous vehicles, intelligent traffic management systems, and predictive maintenance, improving road safety and efficiency.
Future Prospects of AI Infrastructure
As AI infrastructure continues to evolve, we can expect significant advancements in various domains. Some of the future prospects of AI infrastructure include:
- Edge AI: Edge AI enables AI systems to be deployed at the edge of the network, reducing latency and improving real-time decision-making.
- Explainable AI: Explainable AI enables AI systems to provide transparent and interpretable results, addressing the critical challenge of trust and transparency.
- Quantum AI: Quantum AI has the potential to revolutionize AI infrastructure by providing unprecedented computational capabilities and solving complex problems that were previously unsolvable.
- Hybrid Intelligence: Hybrid intelligence enables the seamless integration of human and AI capabilities, enhancing decision-making processes and innovation.
Conclusion
In conclusion, AI infrastructure is the backbone of the next generation of artificial intelligence, providing a robust foundation for AI systems to learn, reason, and interact with their environment. As AI infrastructure continues to evolve, we can expect significant advancements in various domains, enabling the development of innovative applications that were previously unimaginable. By understanding the intricacies of
Oksana Glamour Official
Tyler Hynes Relationships
Jackoherty Girlfriend
Article Recommendations
- Zhao Lusi
- Keean Johnson
- Who Is Brian Adams Partner
- Cassie Parents
- Lyna Perez
- Diddy
- Sophie Raind
- Piddy List
- Karoline Leavitt Husband
- Oprah Andiddy
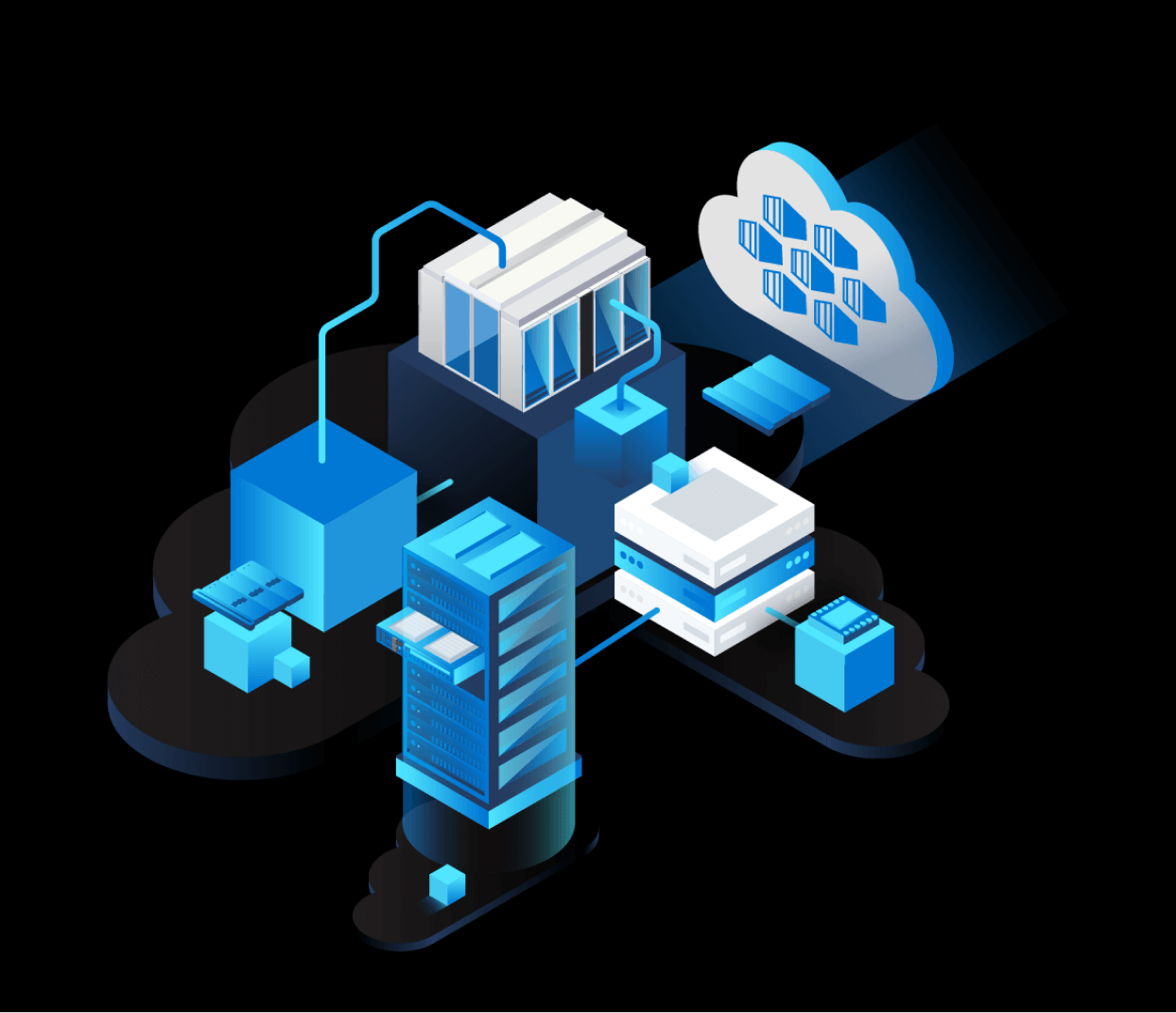
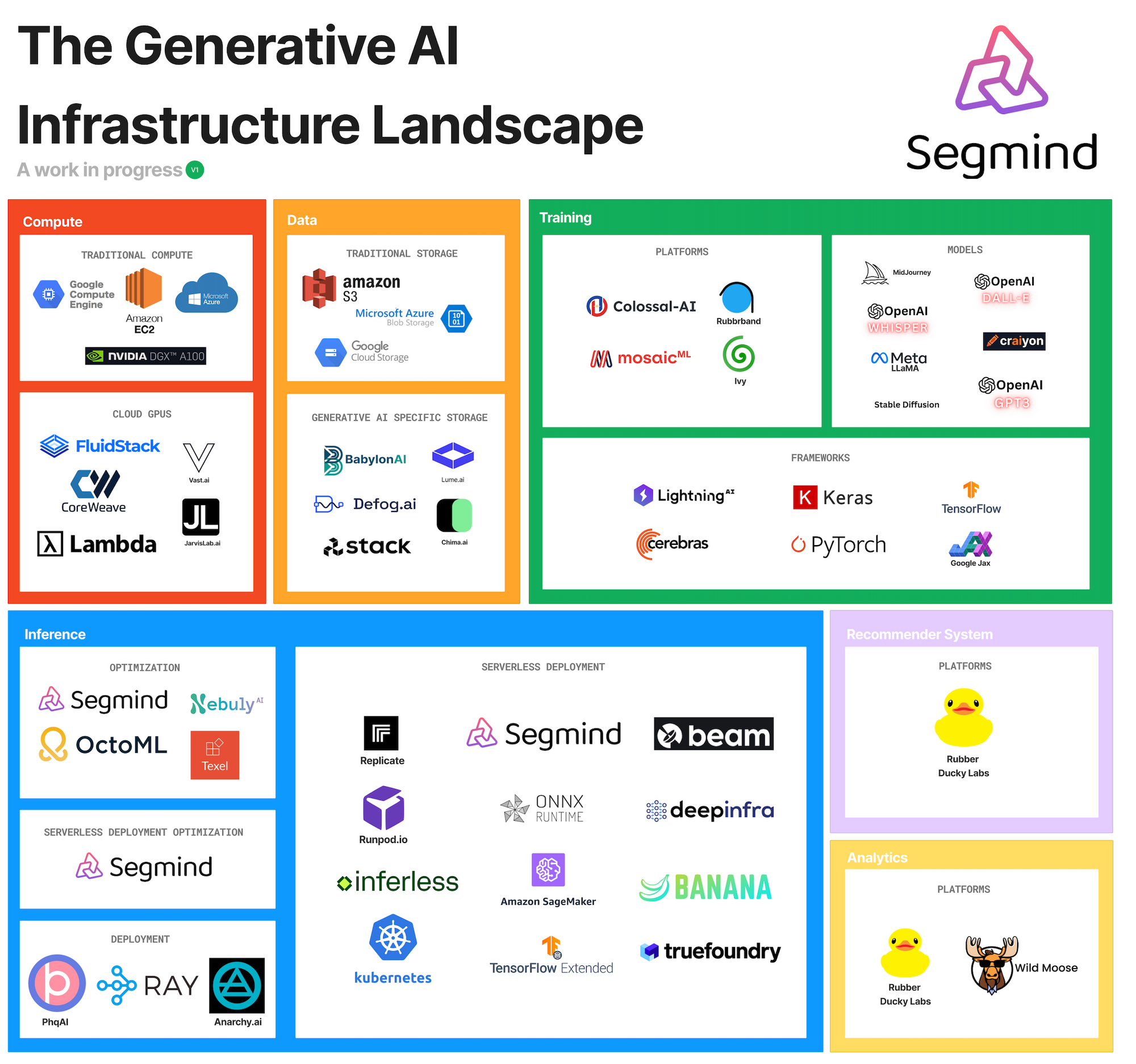
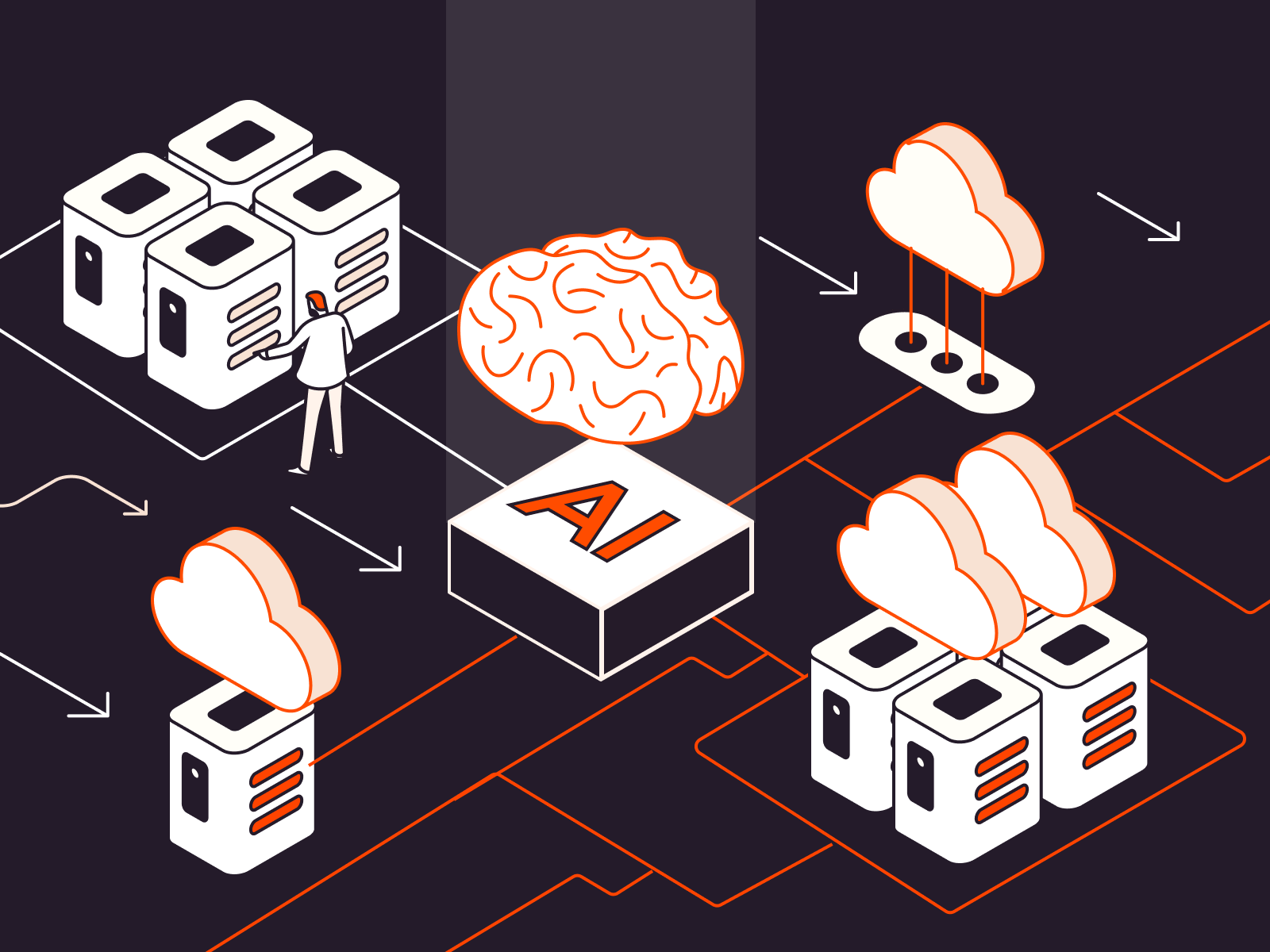