Unveiling the Hidden Worlds of AI: The Secret Datasets Powering the Next Generation of Intelligent Machines
The world of artificial intelligence (AI) has witnessed an unprecedented boom in recent years, with innovations in machine learning, deep learning, and natural language processing leading to groundbreaking applications in various industries. However, a critical component of AI's success remains shrouded in mystery – the vast datasets that power its brain-like functionality. Recent investigations by The New York Times have revealed a staggering array of secret datasets that are transforming the way AI systems learn, interact, and make decisions. This article delves into the fascinating world of these hidden datasets, exploring their significance, usage, and potential impact on the future of AI.
The Importance of Data in AI Development
AI systems rely heavily on vast amounts of data to learn, adapt, and improve their performance. This data can come in various forms, including text, images, audio, and video. The quality, quantity, and diversity of this data have a direct impact on the accuracy, relevance, and effectiveness of AI models. In recent years, the demand for high-quality data has led to the development of specialized data platforms and services that provide access to curated, anonymized, and protected datasets.
Some of the key drivers of the data-driven AI revolution include:
- Lingua Facta: A dataset of over 30 million pieces of text, created by the language learning platform Duolingo, which is used to train AI models to recognize and generate human-like language patterns.
- Stanford's Visual Speech Recognition Dataset: A collection of audio and video recordings of human speech, annotated with detailed transcriptions and lip movements, used to improve AI systems' ability to recognize and transcribe spoken language.
- Google's Street View Data: A vast repository of street-level imagery, capturing the visual landscape of cities and towns worldwide, used to train AI models to recognize and navigate complex environments.
The Types of Datasets Used in AI Development
A wide range of datasets are used in AI development, each with its unique characteristics and applications. Some of the most commonly used datasets include:
- Medical Imaging Datasets: Collections of medical images, such as X-rays, CT scans, and MRIs, used to train AI models to diagnose and detect diseases.
- Social Media Datasets: Archives of social media data, including text, images, and audio, used to train AI models to recognize and generate human-like language patterns.
- E-commerce Datasets: Collections of e-commerce transaction data, including product descriptions, customer information, and purchase history, used to train AI models to predict customer behavior and optimize marketing campaigns.
The Secret Locations of These Datasets
The locations of these secret datasets are often shrouded in mystery, with many datasets being stored in secure, proprietary repositories. Some of the most prominent data hubs include:
- Cloud Storage Services: Cloud storage services, such as Amazon S3 and Google Cloud Storage, provide secure and scalable storage for large datasets.
- Data Lakes: Data lakes are centralized repositories that store large amounts of raw data, often in its native format, allowing for efficient data processing and analysis.
- Proprietary Databases: Proprietary databases, such as those used by the US Census Bureau and the National Institutes of Health, provide secure and controlled access to sensitive data.
The Impact of These Secret Datasets on AI Development
The use of secret datasets in AI development has significant implications for the field, including:
- Improved Model Accuracy: The use of high-quality, diverse datasets has led to significant improvements in AI model accuracy, enabling applications in areas such as medical diagnosis and image recognition.
- Enhanced Transfer Learning: The use of pre-trained models, trained on large datasets, has enabled the development of transfer learning techniques, allowing AI models to adapt to new tasks and domains with greater ease.
- Increased Data Sharing: The development of data platforms and services has facilitated data sharing and collaboration among researchers and organizations, accelerating the pace of AI innovation.
Conclusion
The secret datasets powering AI's brain-like functionality have become a hot topic of discussion in recent years. The recent revelations by The New York Times have shed light on the importance of data in AI development, highlighting the role of specialized data platforms and services in providing access to curated, anonymized, and protected datasets. As AI continues to evolve and improve, it is essential to understand the impact of these secret datasets on the field, and to develop strategies for harnessing their power to drive innovation and progress.
By uncovering the hidden worlds of AI, we can unlock new possibilities for AI development, and create a brighter future for intelligent machines.
Katy Lane Newcombe
Billieilish Y Pics
Google Places Rank Tracking
Article Recommendations
- Rami Malek And Portiaoubleday
- Alma Powell Cause Ofeath
- Barron Trump Heightisease
- Brooke Monkd
- Amariah Moralesd
- Grace Charis Fans
- My Pillow Net Worth
- Michael Boulos Net Worth
- Myerz
- Ava Baroni
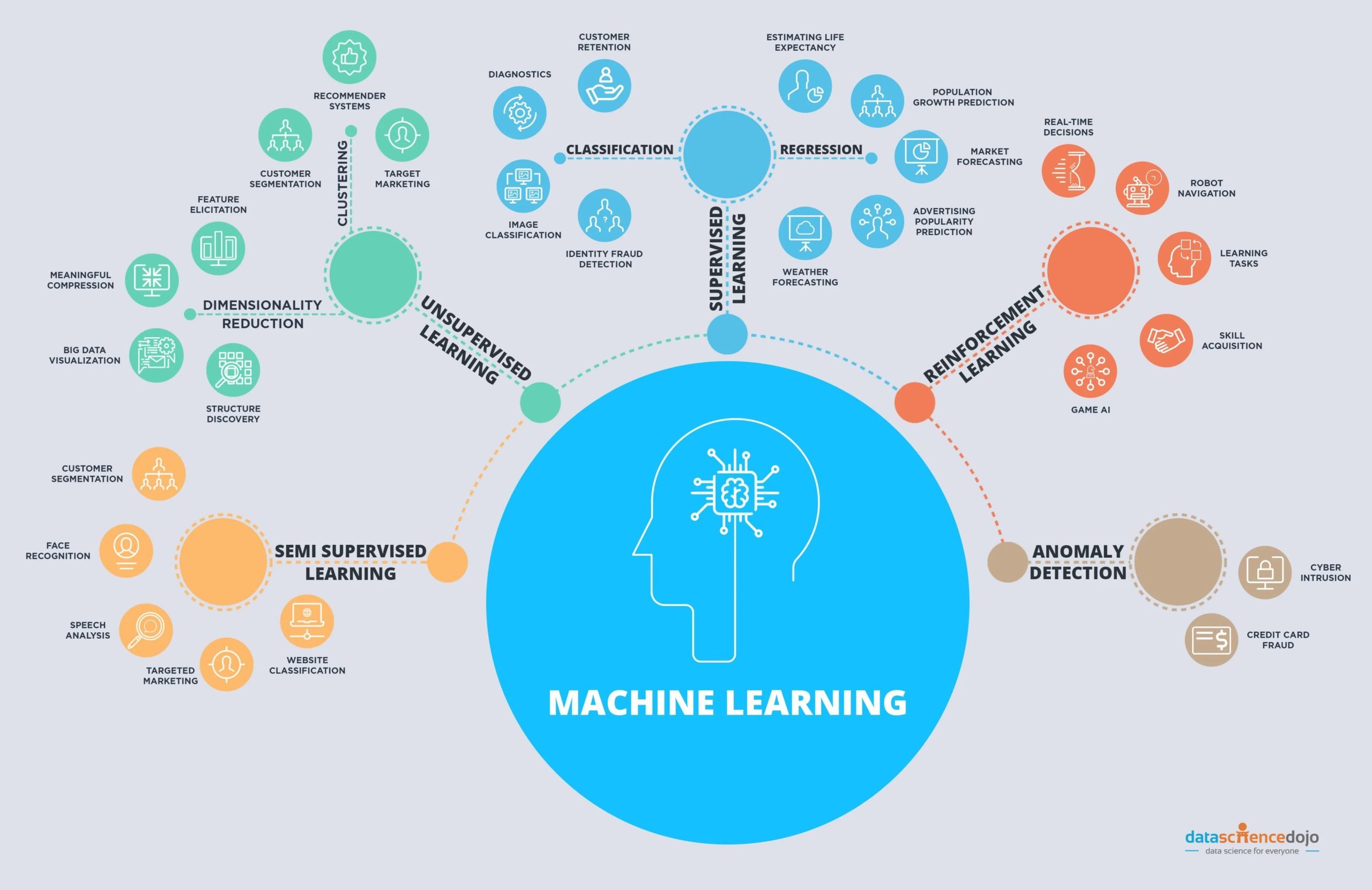
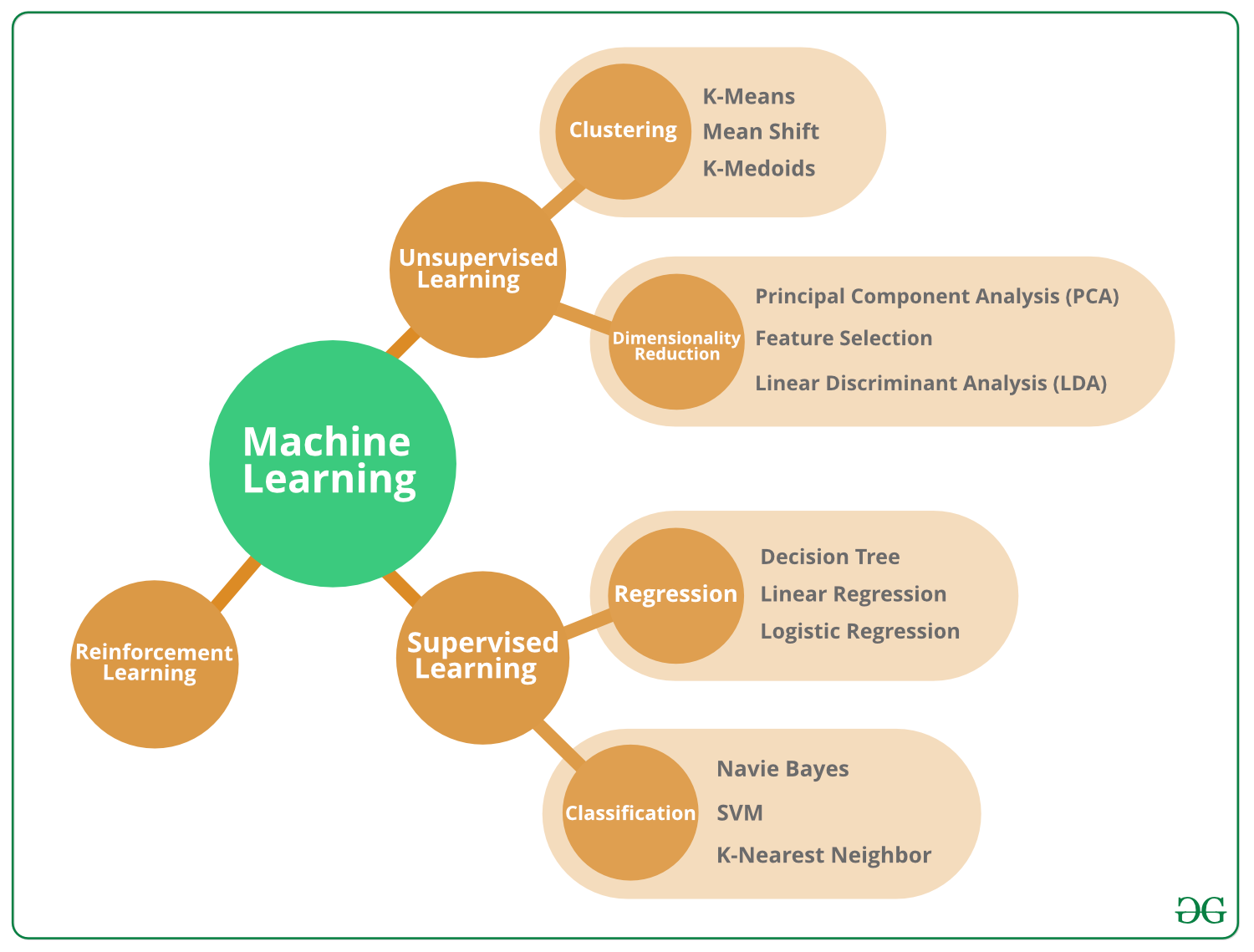
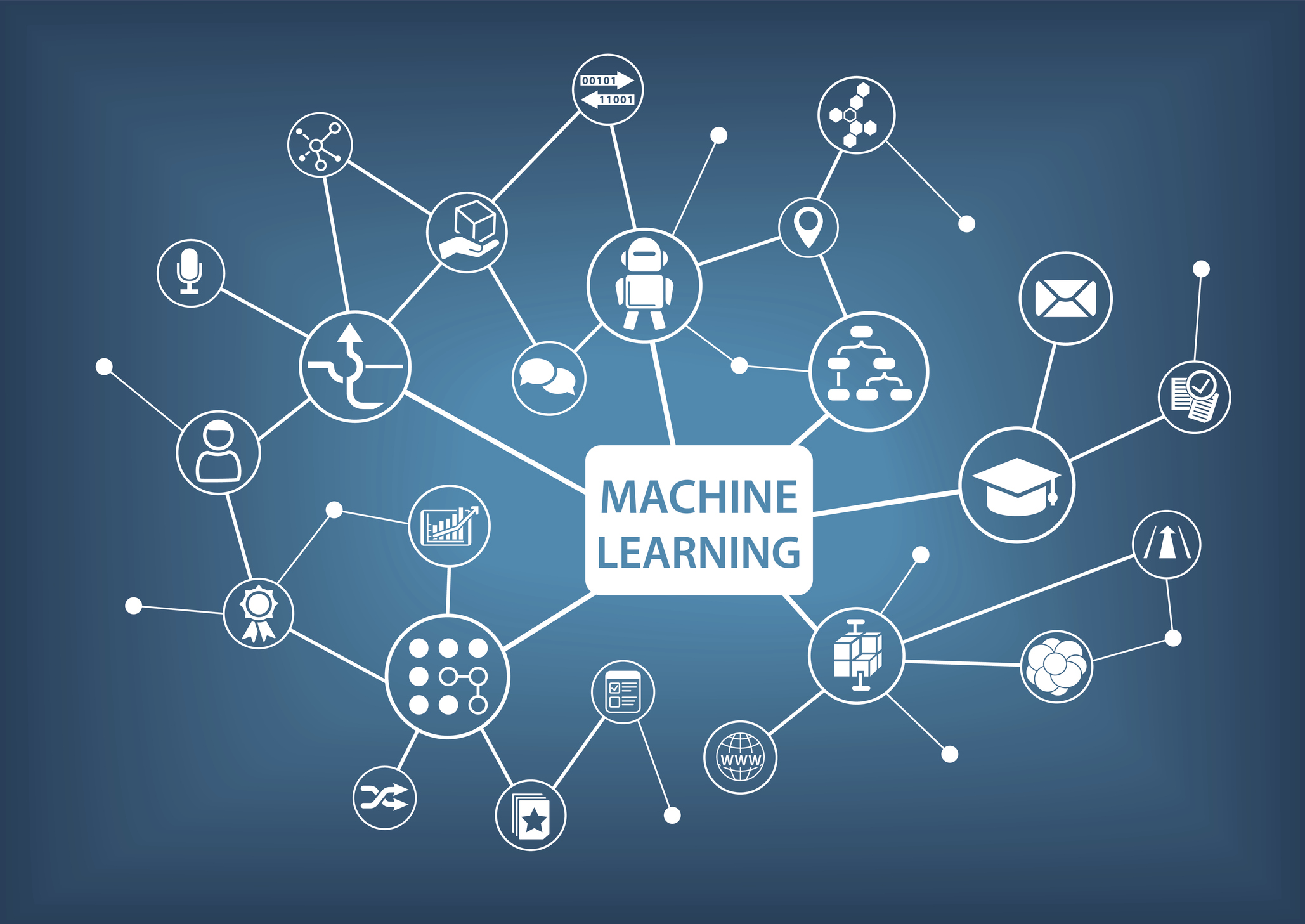